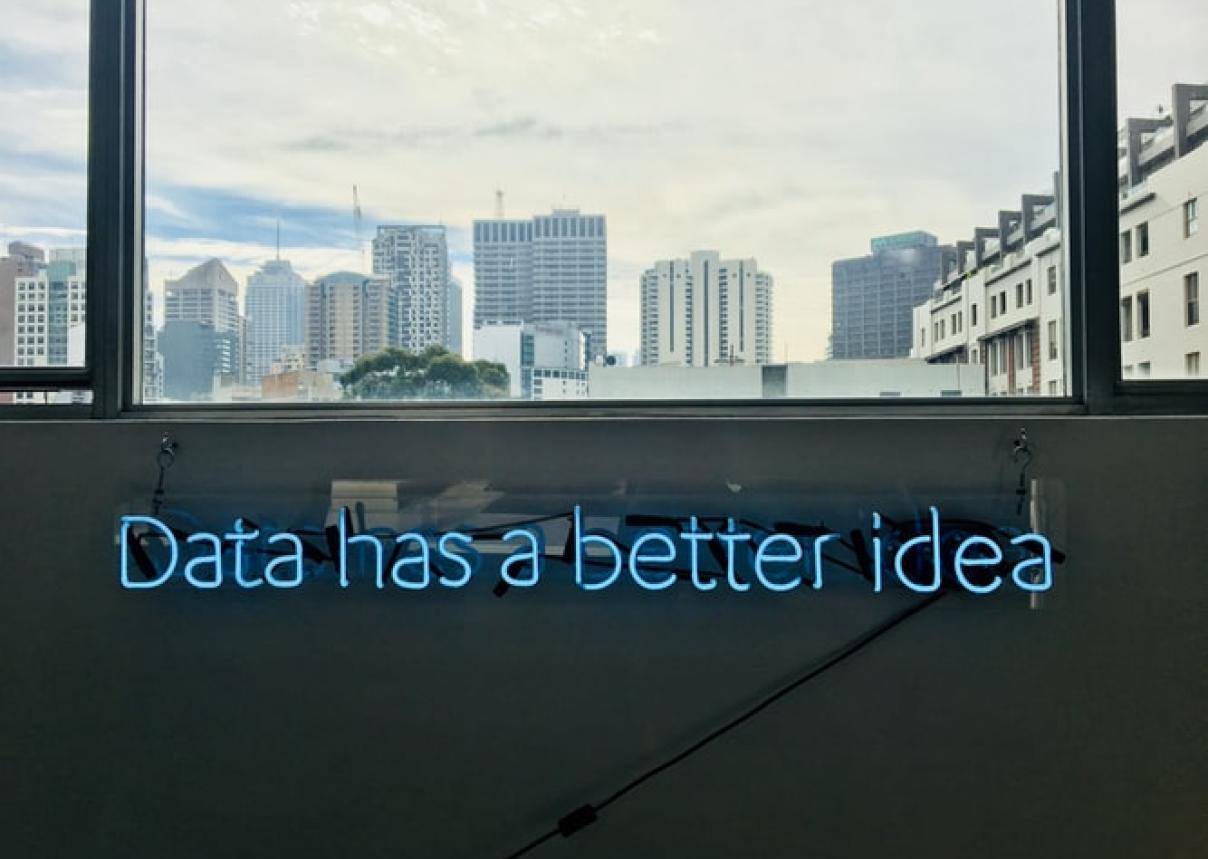
Artificial intelligence is not such a new idea as many think. It’s long been a dream or nightmare in science fiction. The term itself was coined in the 1950s. There’s been talk of ethics round it, too, for more than half a century.
But debates on this today are different, for several reasons. AI systems that affect our lives are now becoming widespread and much more pervasive ones seem imminent, not distant. Businesses and governments are enthusiastic advocates of AI’s potential, while many citizens are anxious. The media love a breakthrough almost as much as they do projected doom. And those breakthroughs seem to come more quickly than had been expected.
Machine learning
Machine learning’s the key issue, in many ways, from an ethical perspective – the ability of systems to improve their ability to perform tasks by learning from big data sets without human intervention; and, as a result, to make decisions without us being able to follow or understand the pathways of decision-making.
That’s uncomfortable if we want to ‘shape the Information Society’ (in the spirit of WSIS), and it poses many questions. Here are three that we should think about:
Ceding decision-making
One – raised widely – concerns the extent to which we’re happy to cede decision-making to machines/technologies/algorithms? We already do this widely, of course, but the extents to which we can and do are growing. And it’s not just obvious decisions that matter; it’s also how we trust and follow recommendations that are made for us by YouTube and the like.
A second – a step further – concerns how far we’re happy for those with power over us (governments, employers, landlords, banks, insurance companies) to cede decisions that concern us to machines/technologies/algorithms? That’s already happening as well and growing rapidly.
The third – another step beyond – concerns not just machine-learning and decision-making, but ways in which those with power over us can use the power of AI and machine learning to reach beyond specific choices (such as who to hire or who should get a loan) to other areas with much greater impact on people’s lives (predictive policing, for example, or ‘reputation management’ like China’s evolving ‘social credit’ system).
So what are ethics for?
Ethics, let’s say, with Google, are ‘moral principles that govern a person's behaviour or … an activity.’ Ethical frameworks are attempts to build consensus around such principles which can be adopted by a community – whether that’s a group of individuals, businesses within the data sector, or the global community of governments and other stakeholders.
Many organisations have been looking to develop ethical frameworks for AI. Naturally, these differ in some respects, but there’s also been a good deal of consensus to them. This week, some general points; next week, some thoughts on one attempt to identify broad principles where that consensus lies.
Why seek an ethical framework?
First questions: what use is a framework? Will it have any influence if it has no legal force? Will it be respected everywhere? Can consensus be agreed between very different ways of looking at the world – between, say, authoritarian and democratic governments, profit-motivated corporations and privacy campaigners, data-managers and data-subjects?
Getting consensus around ethical principles is obviously difficult, but possible (and requires compromise). Securing respect for principles that are agreed is much more difficult than achieving that consensus. Look about you: there are always many – governments, businesses, citizens – whose interests lie in ignoring or undermining agreed frameworks of rights and principles, and who act accordingly.
But it’s generally agreed that frameworks are valuable in two respects. They set a basis that has moral authority: those who abuse or ignore them often feel, at least, that they have to justify themselves, and their critics have a platform from which they can cry foul. This does have positive effects.
And they can help by building global standards which reduce the risks that come with new technologies. Think how important that is, for example, in other high-tech areas like gene-editing.
Why not just use the rights regime?
Next question: what’s new here? Why, some people ask, don’t we just rely on the international rights regime – the Universal Declaration of Human Rights and other rights conventions?
Because they’re a floor that has no detail. They provide the foundation for ethical frameworks as we try to navigate the complex realities of economy, society, culture and technology, but not the scaffolding.
What does the International Covenant on Civil and Political Rights say, for example, about one of the most important issues in the digital society?
‘No one shall be subjected to arbitrary or unlawful interference with his privacy, family, home or correspondence, nor to unlawful attacks on his honour and reputation.’ That’s all it says on privacy. We all know the meaning here’s contested, too, which is why it’s been the subject of elaboration and development by the UN’s Human Rights Council, special rapporteurs, regional rights organisations, governments and others.
The nature of data gathering in the digital society – data gathering by default – is radically different from the past. The scope and capabilities of data analysis – ‘big data’ analysis, so called – reach far beyond anything considered possible just ten years ago.
If there is to be moral authority or global consistency underpinning this, it needs to be in depth, developed now, with widespread expertise, seeking to address present and future realities – building on rights and other agreements that have relevance, yes, but also current and much more extensive. There’s nothing new in this. We’ve been doing it in other areas – genetics, climate change, etc. – throughout the time since governments signed up to rights in 1948.
Algorithms and data
Final question, for the moment: what’s the core of the dilemma? I’ll mention one example here. There are others, some of which I will come back to next week.
The starting point is technical. Artificial intelligence and machine learning rely on two things: algorithms and data.
The algorithm’s the sequence of rules or instructions that an automated system (or indeed the human official that system may replace) follows when making a decision. The data’s the evidence base that is available to that automated system (or indeed human official) to guide what a decision ought to be. Both can be flawed.
An algorithm may be skewed, deliberately or not, with good reason or without, in ways that seek to achieve particular outcomes – for example, towards greater caution in offering loans, or (let’s imagine) prioritising those with higher ‘social credit’ scores.
Data that are currently available will almost certainly be skewed because of the incompleteness and inaccuracy of existing data-sets and past decision-making. As a result, biases in existing data and decision-making are likely to be perpetuated, even exacerbated, by automated systems. Machines that learn on biased data will make biased decisions.
There are already plenty of examples of this. Automating job-hiring on the basis of historic data, for example, has been shown to perpetuate ethnic and gender stereotypes. Law enforcement outcomes have been equally flawed.
Five real-world difficulties
Rectifying this and similar problems is far from easy and is going to run into a number of real-world difficulties. Here are five, each of which could form the subject of a future blog.
-
Many people place more faith in automated decisions than in human ones, believing that they’re more objective (though the data’s biased).
-
Many decisions affecting people’s lives require nuanced response to individual circumstances as well as general fairness (particularly where they concern vulnerable individuals and communities with complex needs).
-
Many of the most valuable data sets are held by businesses whose priority is to monetise their value, affecting the ways in which they feed into both their own and governments’ big data analysis.
-
Many governments are concerned to reduce the cost of government, which encourages them to rely on automated systems without sufficient safeguards against bias or protections for individuals.
-
Many actors who’ll make use of automated systems will seek to exploit them for their own (economic, political or other) advantage rather than to maximise their value for the public good (‘AI for Bad’ is as important as ‘AI for Good’.)
These are real world challenges. Ethical frameworks for new technologies are intended to help maximise the potential benefits and minimise potential harms around them.
Next week, I’ll look at one suggested set of principles to do this.
Image source: Franki Chamaki, www.hivery.com via Unsplash.